Artificial intelligence predicts response to cancer therapy based on data from each tumor cell
Sist anmeldt: 14.06.2024
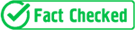
Alt iLive-innhold blir gjennomgått med medisin eller faktisk kontrollert for å sikre så mye faktuell nøyaktighet som mulig.
Vi har strenge retningslinjer for innkjøp og kun kobling til anerkjente medieområder, akademiske forskningsinstitusjoner og, når det er mulig, medisinsk peer-evaluerte studier. Merk at tallene i parenteser ([1], [2], etc.) er klikkbare koblinger til disse studiene.
Hvis du føler at noe av innholdet vårt er unøyaktig, utdatert eller ellers tvilsomt, velg det og trykk Ctrl + Enter.
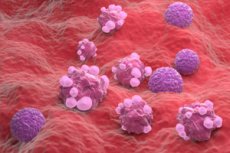
With more than 200 types of cancer and each individually unique case, ongoing efforts to develop precision oncology treatments remain challenging. The focus is on developing genetic tests to identify mutations in cancer driver genes and identifying appropriate treatments against these mutations.
However, many, if not most, patients with cancer do not benefit significantly from these early targeted therapies. In the new study, published in Nature Cancer, first author Sanju Sinha, Ph.D., assistant professor in the Molecular Therapeutics Program in Cancer at Sanford Burnham Prebys, together with lead authors Eitan Ruppin, MD, PhD, and Alejandro Schaffer, PhD, from the National Cancer Institute, part of the National Institutes of Health (NIH), and colleagues describe a unique computational system for systematically predicting patient response to cancer drugs at the single-cell level.
Called Personalized Treatment Planning in Oncology Based on Single-Cell Transcript Expression (PERCEPTION), the new artificial intelligence-based approach delves into the study of transcriptomics—the study of transcription factors, the mRNA molecules that are expressed by genes and translate DNA information into action.
"A tumor is a complex and constantly changing organism. Using single-cell resolution allows us to solve both of these problems," says Sinha. “PERCEPTION enables the use of rich information from single-cell omexis to understand tumor clonal architecture and monitor the emergence of resistance.” (In biology, omexis refers to the sum of the constituents within a cell.)
Sinha says: "The ability to monitor the emergence of resistance is the most exciting part for me. This has the potential to allow us to adapt to the evolution of cancer cells and even change our treatment strategy."
Sinha and colleagues used transfer learning, a branch of AI, to create PERCEPTION.
"Limited cell-level data from clinics was our main challenge. AI models need large amounts of data to understand disease, just like ChatGPT needs huge amounts of text data from the Internet," explains Sinha.
PERCEPTION uses published bulk gene expression data from tumors to pretrain its models. Next, single-cell level data from cell lines and patients, although limited, were used to tune the models.
PERCEPTION was successfully validated in predicting response to monotherapy and combination therapy in three independent, recently published clinical trials in multiple myeloma, breast and lung cancer. In each case, PERCEPTION correctly stratified patients into responders and non-responders. In lung cancer, he even documented the development of drug resistance as the disease progresses, which is a significant discovery with great potential.
Sinha says PERCEPTION is not yet ready for use in the clinic, but the approach shows that information at the single-cell level can be used to guide treatment. He hopes to encourage the adoption of this technology in clinics to generate more data that can be used to further develop and improve the technology for clinical use.
“The quality of forecasting improves with the quality and quantity of data on which it is based,” says Sinha. "Our goal is to create a clinical tool that can systematically and data-drivenly predict treatment response in individual patients with cancer. We hope that these findings will stimulate more data and similar studies in the near future."